Real-world data (RWD) holds immense promise for advancing medical research and accelerating the development of new treatments. With regulatory agencies like FDA increasingly encouraging the use of RWD for primary evidence in submissions, the opportunity to leverage these data sources for clinical trials and regulatory applications is more significant than ever. However, tapping into this potential isn't without challenges—the journey from raw RWD to reliable insights faces a huge hurdle to prove accuracy and trustworthiness of the generated evidence.
In a talk at the DIA Real-World Evidence (RWE) conference in October 2023, Mayur Saxena, CEO of Droice Labs, explored the intricacies of this issue through a hypothetical scenario in which a team of scientists is attempting to use RWD to accelerate a clinical trial through an external control arm (ECA). Following their journey, this talk shed light on how and why the processing of RWD for research leads to unreliable data and what can be done about it. The talk prompted a discussion in the audience by FDA representatives and other stakeholders that highlighted that FDA’s requirements for data reliability will not be lower for RWD, and that the industry needs to focus on rigorous scientific approaches to ensure the trustworthiness of study inferences when using RWD. This article tells this story.
Leveraging RWD to Accelerate Primary Evidence Generation
Imagine a team of scientists working to develop a groundbreaking drug aimed at preventing strokes in patients with atrial fibrillation (AFib). To accelerate their research, they decide to set up an ECA using RWD to support an FDA submission. They plan to gather data from 50,000 patients across 20 hospitals around the world. These patients for the study should all be receiving the standard of care—direct oral anticoagulants (DOACs).
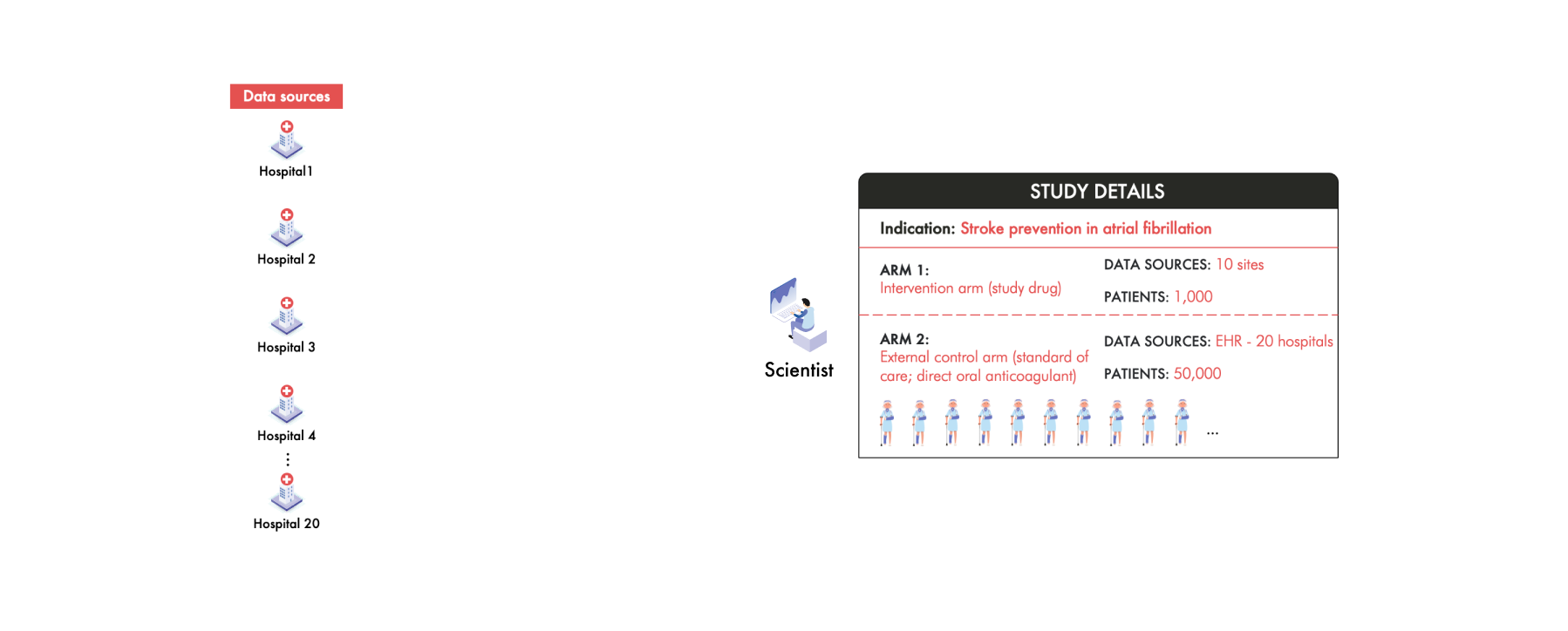
The Challenge of Diverse RWD Sources
The challenge in building this ECA however, is that each of these 20 hospitals collects and stores patient data differently. For example, Hospital 1 uses an Epic electronic health record (EHR) system while Hospital 3 uses Cerner. Between these two sources the data is collected into different structures and the patient clinical information is represented differently. Even hospitals using the same EHR vendor can have significant variations due to unique clinical workflows and customization.
Take Hospital 20 as an example. Like Hospital 1, they have Epic, but to improve efficiency they've adopted a system where patients input their current medications into an app while waiting to see their clinician. This data is collected differently from the medication data in Hospital 1 and is stored differently as well. For each of the 50,000 patients across these hospitals, their medication information is scattered in various places and in different formats within each hospital's EHR system. For example, in this study, the scientists find that Patient 1’s medication data is stored in 12 different places within EHR 1, Patient 2’s in 16 places within EHR 2, and Patient 236’s in 23 places in EHR 20.
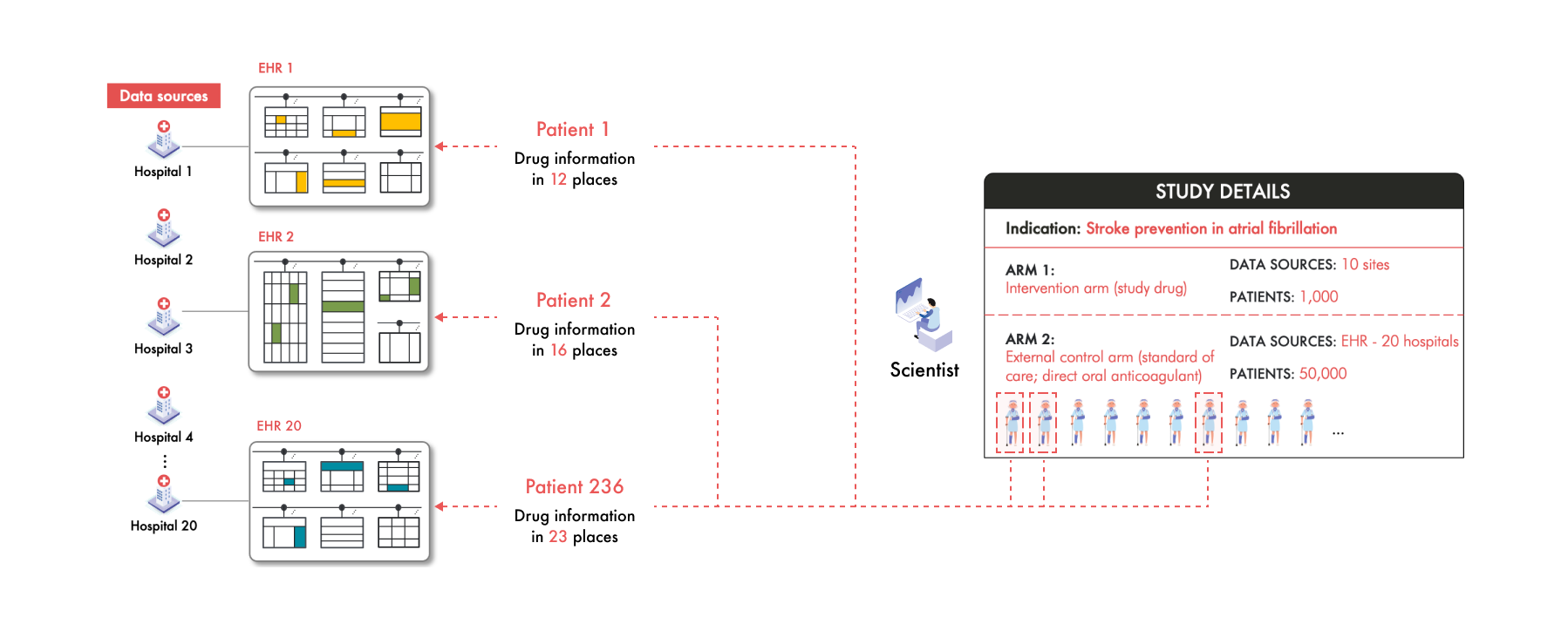
But these scientists aren't just looking for medication data—they need a plethora of clinical information—diagnoses, lab results, vital signs, and many more. Navigating through 20 different data structures to extract this information is not only cumbersome but practically unmanageable. Multiply this complexity across the diversity of coding systems of clinical concepts and the unique way each of the thousands of patients’ clinical information manifests and the challenge becomes monumental.
Querying and analyzing data from these diverse structures and coding standards is therefore an enormous task that’s nearly impossible to manage without a unified system. This is where the common data model (CDM) comes into play.
Common Data Models: a Solution to Organize and Harmonize RWD
To address this complexity, the scientists turn to a Common Data Model (CDM). This CDM provides a standardized data structure where specific types of information are stored in predefined tables or fields to facilitate analysis. For example, all drug information will be consolidated into a single "Drug" table, all of the labs into the “Labs” table, and all the diagnosis codes into the “Conditions” table.
By mapping and transforming the disparate data from each hospital into a single CDM, all this data from all 20 hospitals is now in one place, in one structure, in one format. For the scientists, this seems like a huge advantage. Instead of dealing with 20 different data structures, they now interact with one unified model, simplifying downstream analytics and potentially accelerating the research process.
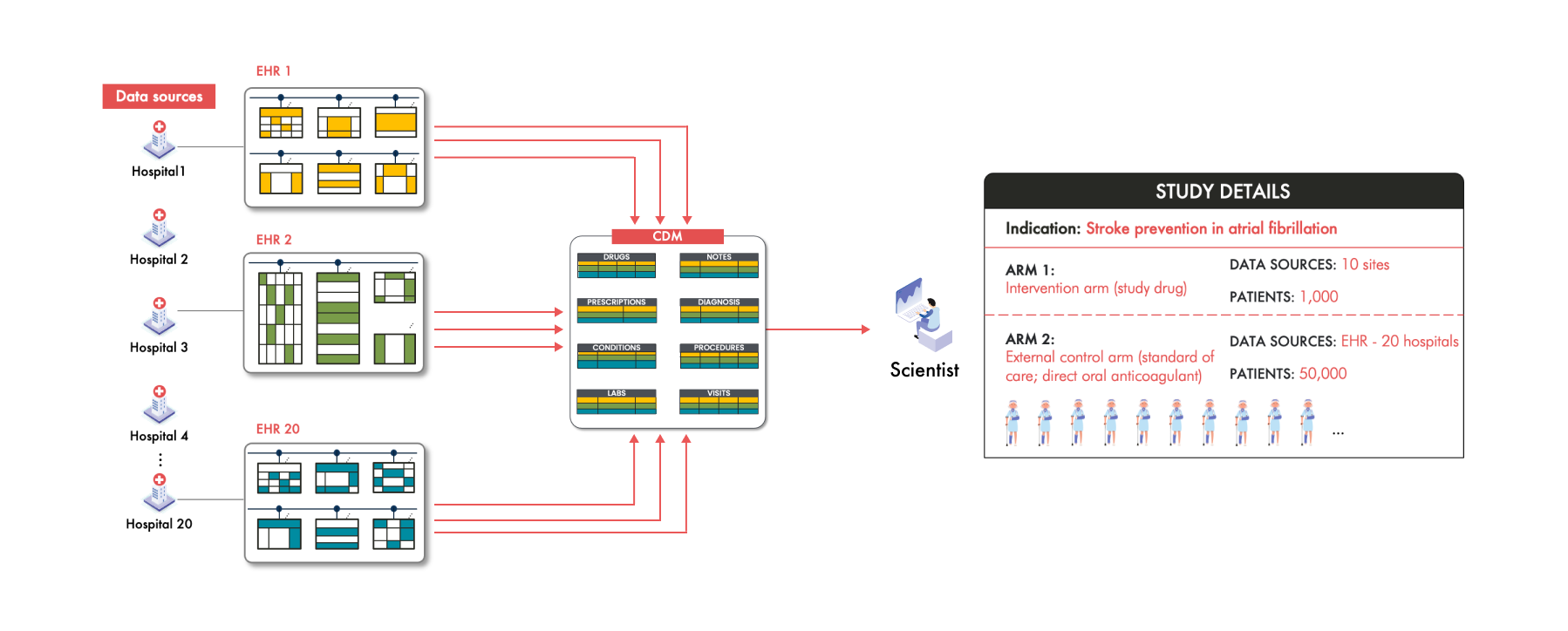
However, this solution isn't without its pitfalls. According to recent FDA guidance on assessing EHR and claims data, when transforming data from multiple sources into a CDM, it's crucial to exercise caution. The FDA notes that it's rare for all the nuanced clinical information from the source data to carry over into the CDM. This phenomenon is referred to as "data loss."
…combining many data sources, especially with the addition of data transformation into a CDM, adds a layer of complexity that should be considered.…
…data in CDM-driven networks rarely contain all of the source information present at the individual health care sites, and the data elements chosen for a given CDM network may not be sufficient for all research purposes or questions…

What Does Data Loss Look Like?
Consider some of the scientists' findings after data transformation of these 20 hospitals to the CDM:
In Hospital 1, only 2% of patients appear to be on DOACs—the expected standard of care
In Hospital 3, only 23% of patients have recorded blood pressure measurements
These are alarming anomalies—these implausibly low numbers indicate something is wrong. Why is this happening?
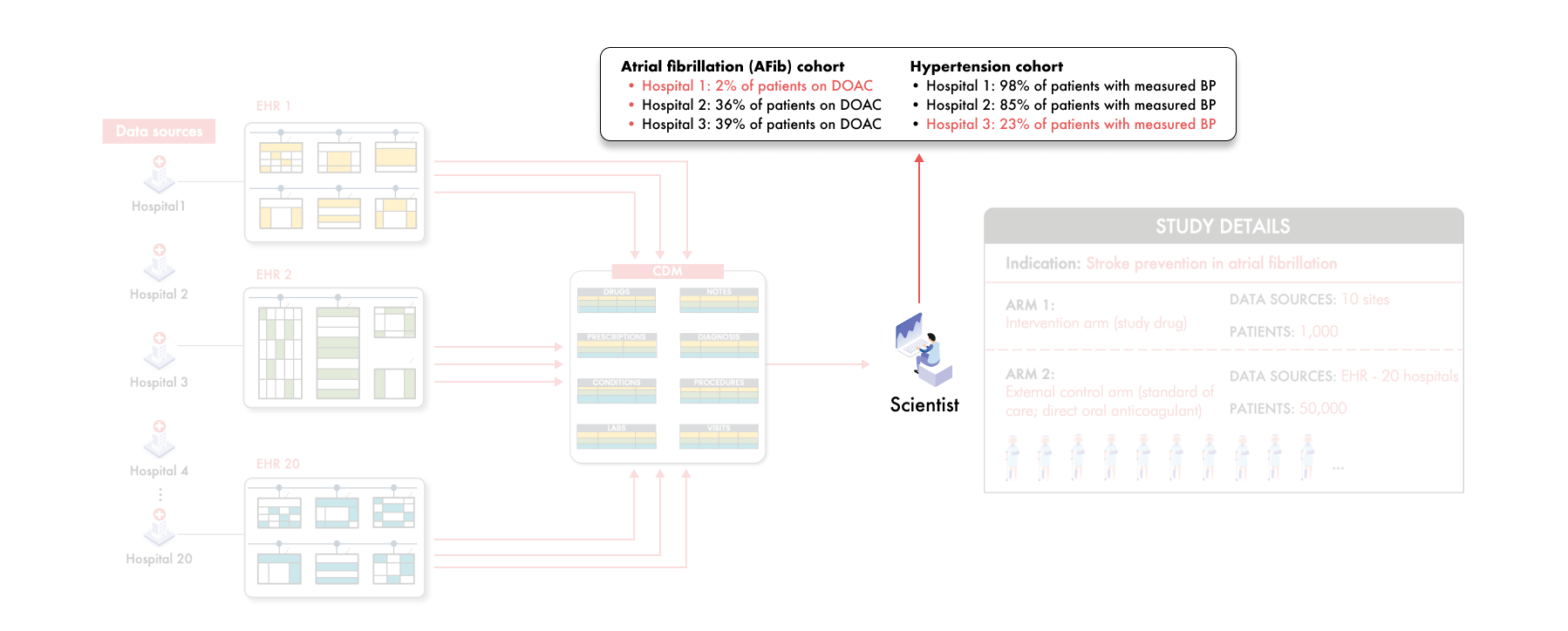
How Data Loss Happens
Let’s put this task to bring RWD to a CDM into perspective. A typical EHR system contains over 10,000 tables that need to be explored to find the relevant data. Multiply that by 20 hospitals, and the number of tables to consider exceeds 200,000. If we add the terminology code mappings and unstructured data elements, the mapping decisions to consider goes from 200,000 to several millions if not tens of millions. How are these mapping decisions made? An army of well intentioned IT professionals at the hospital and/or data vendor have to sift through these diverse, massive, and complex data structures of each hospital's EHR system to harmonize the RWD into a single, standardized structure. Human error is inevitable in such a colossal mapping exercise.
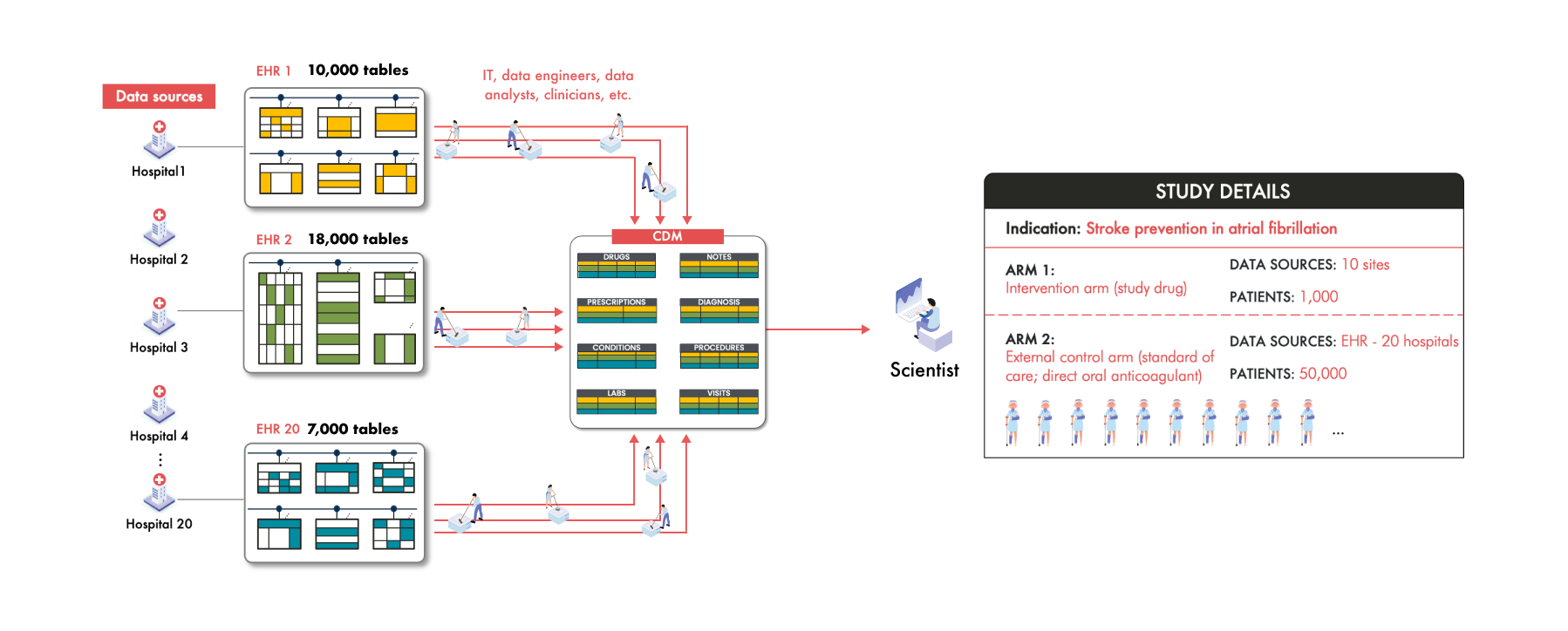
Even if errors are made in mapping just 0.01% of these tables or terms, that still amounts to mistakes in 20 tables and/or 1000s of codes. Furthermore, the impact of data loss isn't proportional to the number of tables or codes missed—it can be far more significant. Missing or incorrectly mapping even a single table or code could result in the loss of all DOAC medication data for patients in a particular hospital.
The Real-World Consequences of Data Loss: Unreliable Data
Coming back to our scenario, these scientists start working with the IT team to find out what went wrong. The IT team went back to search for the missing DOAC data, and now they find 36% of the AFib patients are on DOACs in Hospital 1. But was this new number accurate, even if it was now more plausible—might it actually be 34%, or 42%? Forget Hospital 1, is the 36% in Hospital 2 really 36%? How do we know?
As the scientists continue to go through the CDM data, more and more anomalies surface across the different hospitals. Could there be more DOAC data still missing, or could some of these patients not actually be on DOAC? Uncertainty persists, casting doubt on the validity of conclusions drawn from the data and causing the scientists to give up on the original goal to use these data as primary evidence in the submission.
What Can Be Done?
So should these scientists keep using a CDM? If they have the option, they should not. However the practical reality is they will have to use it—most data that pharma companies have access to will come in a CDM. Given these data quality challenges, how can these scientists trust the data in their CDM? The answer lies in treating this transformed data as an output that requires scientific validation—much like any other experimental result. This involves validation.
For every data point the CDM indicates as positive—such as a patient being on a DOAC—it must trace back to the source data, to the exact evidence that supports this fact, to be verifiable as true. Furthermore, the CDM must also be comprehensively evaluated for false negatives, ensuring that the absence of data isn't due to transformation errors or omissions. Quantifying this error, knowing whether the error bound is 2%, 5%, or even 50%, is crucial to understand the reliability the study inferences. This is exactly what FDA is looking for to demonstrate the reliability of RWE.
...
When converting multiple data sources into a CDM, processes used for data transformation into a CDM (e.g., common terminology and structure), the comprehensiveness of the CDM (e.g., does the CDM contain the key data elements)...

Droice Labs Technologies: Making RWD Reliable
RWD is a gold mine of insights if it can be reliably used, offering the potential to reduce required enrollment in clinical trials and bring life-changing therapies to market faster. But working with raw RWD is really, really hard. Utilizing CDMs is an important way to work with these data as they are extremely useful for scaling the analysis for wide-ranging research questions. However, health systems and physicians prioritize patient care over data entry with research in mind, which is as it should be. This means that the transformation process of these diverse, complex RWD sources into a CDM—involving many thousands to millions of decisions for each source—will always involve some level of error and data loss no matter how perfect the CDM is. This does not mean that CDMs should not be used by these scientists—they just need to recognize these inherent challenges of data loss and treat the CDM as an experimental result that requires rigorous scientific validation.
And this is exactly what Droice SuperLineage enables, providing comprehensive traceability for all source data elements—a critical FDA requirement for data reliability and validation (read about Droice’s discussions on SuperLineage with FDA).
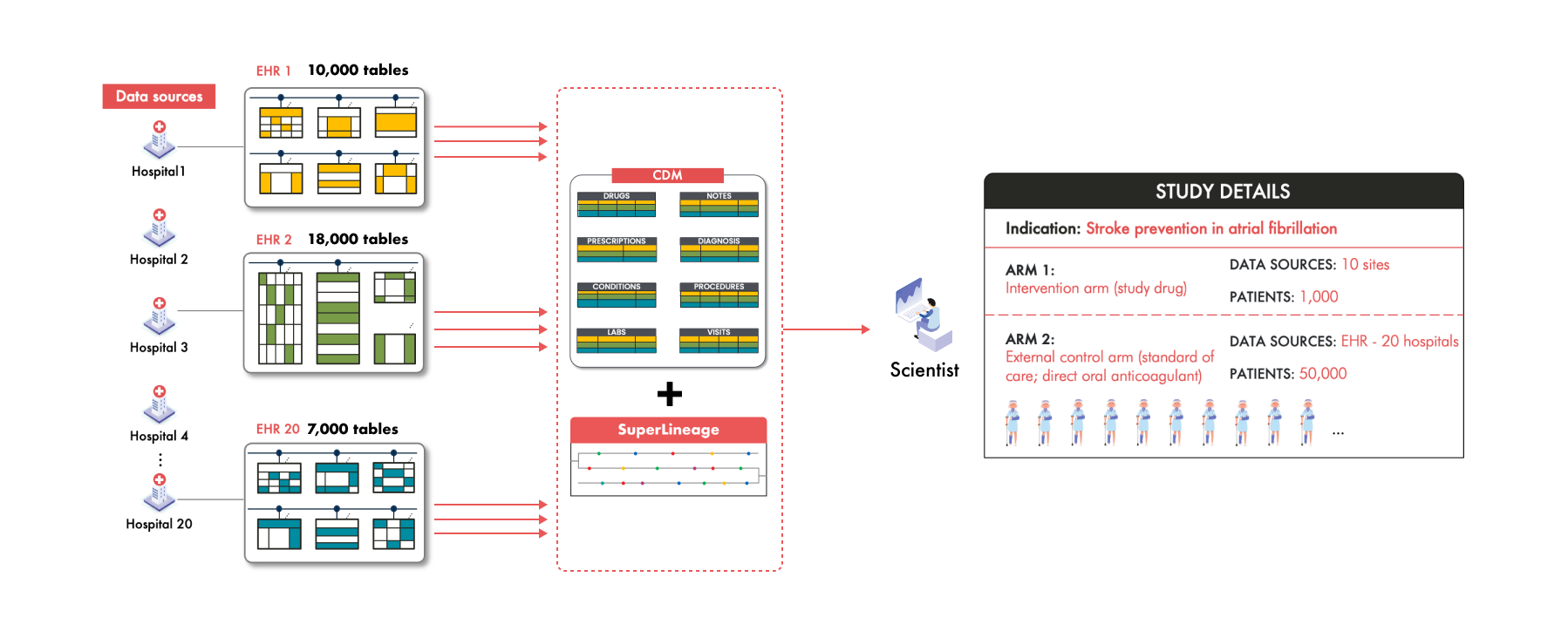
Droice Hawk is an AI middleware, trained on massive volumes of global RWD, that scalably converts raw, messy RWD into reliable analysis-ready data, accelerating data processing for RWD-driven studies by orders of magnitude. These dedicated AI technologies solve these exact data loss challenges involved in taking RWD to CDMs. Together, these technologies have helped pharma meet FDA requirements for “accuracy, completeness, and traceability”. Learn more: www.droicelabs.com.