Blog
Edited by Brian Gillette, Tasha Nagamine
Introduction
At DIA Global 2024, industry leaders in the real-world evidence (RWE) field Julia Luan (AstraZeneca), Victoria Chia (Amgen), and Hongwei Wong (Abbvie) participated in a panel discussion about leveraging real-world data (RWD) to streamline clinical trials, organized and chaired by Mayur Saxena, CEO of Droice Labs. The panel dug deep into the practicalities of working with RWD in clinical trials and gave insights into some of the key lessons learned from both successes and failures of using RWE in regulatory submissions.
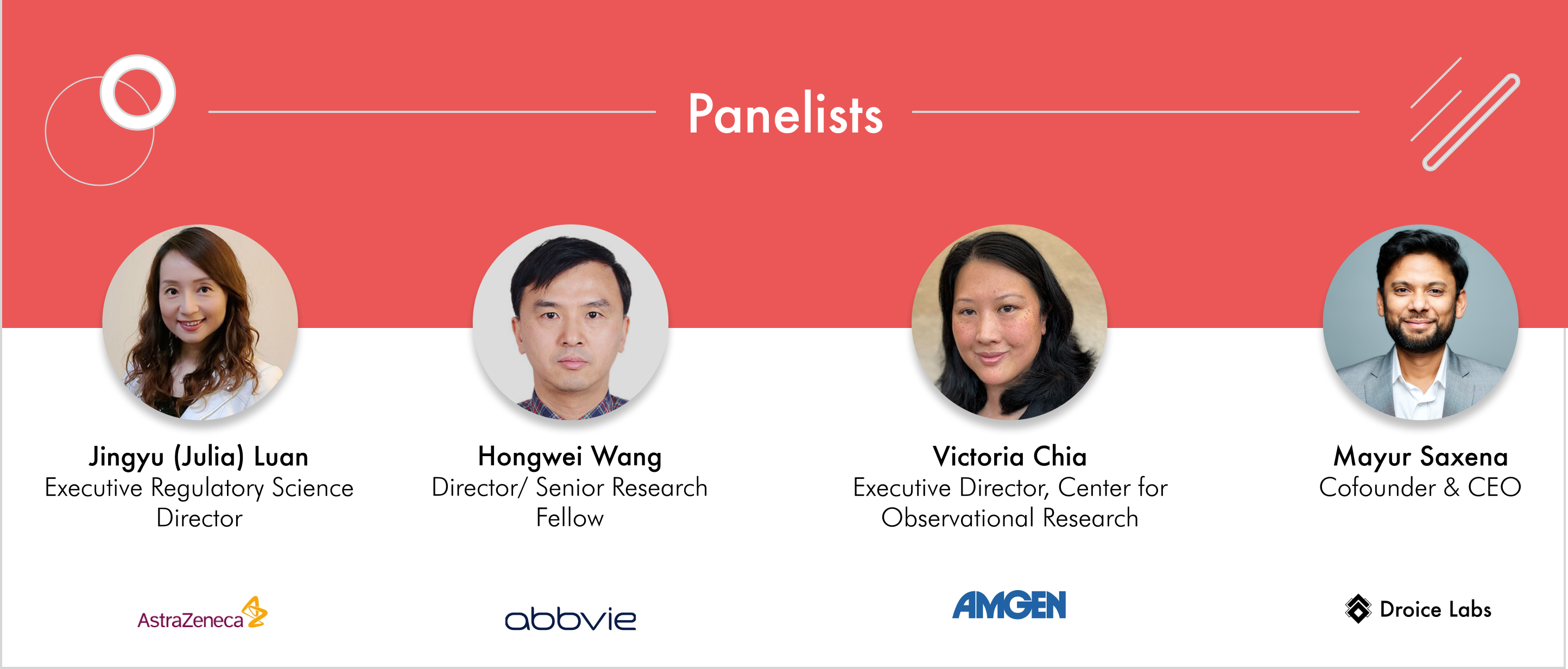
Challenges in regulatory use of RWD
Because of the many challenges involved in using RWD to derive valid, reliable insights about drug efficacy and safety, the majority of RWD used in regulatory submissions to date has been for supportive or secondary evidence. However, FDA has been raising the bar for demonstrating scientific rigor and data reliability for any RWD used in regulatory decision-making, whether for primary or supporting evidence.
Why is RWE being rejected by FDA?
Mayur brought up the fact that RWE included in pharma FDA submissions has been frequently rejected or not considered in regulatory decisions and asked the panel to share their insights into the reasons why this is happening.
Julia, who was an FDA reviewer in the CDER office of biostatistics for 13 years before leading the global regulatory strategy team at AstraZeneca, shared insights from a review of FDA submissions using RWD ECAs that she coauthored with a group of former FDA statisticians that had extensive experience in reviewing biologics licensing applications (BLAs) and new drug applications (NDAs) using RWD. She explained that the paper summarizes lessons learned for 20 notable FDA submissions in oncology and rare diseases that used RWD ECAs to support drug efficacy and/or safety. Their review of the application packages revealed that for approximately half of the applications that submitted ECAs, the ECA data was not even considered in the regulatory evaluation and was not included in the label. The major reasons for lack of acceptance of the RWD in the labeling involved RWD reliability or relevance issues, including:
- Issues with comparability of how the variables were assessed in the ECA versus the CT arm, particularly how the criteria were defined for the population inclusion/exclusion criteria, index dates, outcomes, follow-up periods, and censoring
- Missing or incomplete information on risk predictors or other key variables
- Lack of prespecification of the analysis that raised the spectre of cherry-picking supportive results
Hongwei and Mayur expanded upon the issues caused by RWD not being “fit-for-purpose”, clarifying that the two components of fitness for purpose that need to be addressed are relevance and reliability:
- Relevance, according to FDA guidance, is data comparability and sufficient availability of required variables, for example:
- If the CT arm is at an academic medical center and the RWD comes from community hospitals, the care might not be sufficiently comparable to justify using that source for the ECA
- If the outcome or other key variable in the CT arm is collected through a method not commonly used in real-world settings, the lack of availability of a comparable variable may be prohibitive
- Reliability, according to FDA guidance, is accuracy, completeness, and traceability
Victoria, who has worked on multiple programs with accelerated approvals at Amgen, and Mayur both highlighted the key importance to regulators of traceability, drawing a parallel to source data verification in clinical trials:
- Traceability is the only way to prove whether an individual patient actually had a given inclusion/exclusion criterion, exposure, covariate, or outcome when using RWD
- Traceability to original source data is critical for measuring the accuracy of study variables, which enables potential bias due to misclassification to be evaluated and accounted for in the study analysis
Mayur explained that FDA now expects quantitative proof of RWD reliability, citing examples of when Droice has seen sponsors succeed through quantitative assessments of accuracy, completeness, and traceability.
What are the differences between RWD and RCT data?
Mayur asked the panelists to address the differences between RWD and clinical trial data and how these differences can contribute to rejections.
Hongwei, a biostatistician at Abbvie who has been leading evidence generation activities across various therapeutic areas for over two decades in pharma, contrasted RWE with traditional RCTs. He explained that RCTs are the gold standard for evidence generation for a reason - they give high confidence in the analysis results through:
- Mitigating bias through randomization
- Precisely controlling the experiment through strict inclusion/exclusion criteria and clinical protocols
- Maintaining data quality and reliability through manual collection and quality assurance (QA)/quality control (QC) processes such as source data verification
However, the downside of RCTs is they are not always generalizable due to the narrow populations and care settings in which they are conducted. By contrast, RWD has the advantages of real-time and long-term availability of information for billions of patients across diverse geographies and care settings like hospitals, clinics, and home care. However, there are other differences from RCTs that make RWD challenging to use, especially because of the potential for biases:
- Lack of masking, randomization, and protocolized care means that provider knowledge of patient conditions and treatments can impact management decisions and surveillance for outcomes
- Missing data that was either not collected or not available in the sources used can bias the distribution of exposures, outcomes, and covariates
- Misclassification of patient variables that results from missing data or transformation errors can obscure the true relationships between exposures and covariates with outcomes
- Differences in the definitions of outcomes or other variables in the trial vs. RWD can limit comparability of the same variable between RCT and RWD
Importantly, Mayur and Hongwei both emphasized these challenges necessitate different methodological approaches to analysis versus RCT that account for these issues, especially quantitative bias analysis and other sensitivity analyses that evaluate the reliability of study inferences given misclassification or changes in variable definitions or assumptions.
How should sponsors engage with regulatory agencies when planning trials using RWD?
Mayur raised the critical importance of FDA Type C meetings for sponsors to discuss and align with FDA on how rigor will be ensured in all aspects of study design and execution when using RWD in clinical trials. Hongwei and Julia suggested that teams should pay close attention to the guidance released by FDA and other regulators, noting that the evidence standards remain the same as expected for RCTs when including RWE in the submission package. Victoria gave some examples of the approach at Amgen, in which several steps are taken even before bringing the protocol to regulators:
- Understanding potential threats to validity that are frequently cited in regulators’ criticisms of external control studies, such as confounding, selection bias, misclassification, and the comparability of exposures and outcomes between the ECA and CT arms
- Determining if the data is fit for purpose; that is, if it is relevant to the clinical trial population, and if the data is reliable, meaning is it accurate, complete, and traceable
- Prespecifying the RWE analysis in the protocol and statistical analysis plan
- Ensuring that the study is feasible and that the required inclusion/exclusion criteria, exposures, covariates, and outcomes can be reliably measured in the RWD
Mayur noted that there is RWD expertise within FDA that can be leveraged in meetings like the Type C meeting with the FDA review divisions to facilitate understanding of RWD-specific protocol issues.
for Observational Research
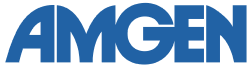
Key takeaways
Accelerating clinical trials with RWD
With this understanding of the key considerations to ensure successful use of RWE in regulatory submissions, the panelists turned to review several approaches that leverage RWD for primary evidence to accelerate trials, including:
- ECAs
- Hybrid controlled trials
- RWD-powered covariate adjustment
What are external control arms (ECAs)?
Mayur asked the panelists to define external controls in clinical trials and discuss some of the benefits of ECAs for clinical trials.
All three panelists were in agreement with the definition of ECAs as control groups created from data external to the trial at hand, noting the following important characteristics of ECAs:
- ECAs can be historical, meaning the external data was generated before the current trial, or the ECA can be concurrent, meaning the ECA patients are undergoing care at the same time as the trial participants
- ECAs can come from RWD sources like electronic health records (EHR), medical claims, and registries, or from past clinical trial data
- ECAs help address situations in which it is difficult or ethically challenging to enroll patients in placebo arms, which is often the case in rare diseases and oncology
- By eliminating enrollment of control patients, overall sample sizes can be significantly smaller in ECA studies, drastically reducing the time and expense of the trial and increasing overall drug development productivity
What are hybrid-controlled trials?
Mayur brought up an emerging new strategy to leverage RWD in RCTs referred to as hybrid-controlled trials, in which only part of the RCT control arm is replaced with external controls. Julia and Hongwei shared their expertise on this approach:
- Hybrid control studies start by enrolling control patients internal to the trial and then add external control patients dynamically, reducing the overall number of internal controls that need to be enrolled compared to the traditional RCT
- The ability to dynamically “borrow” external controls is enabled by established methods to evaluate and ensure the comparability of the external and internal controls
- Hybrid-controlled designs bring the benefit of reducing the number of control patients that need to be enrolled while mitigating the risks of potential bias and insufficient comparability of a fully external control arm
Can ECAs be used in common diseases?
Mayur noted the fact that to date, most regulatory submissions using ECAs have been in rare disease and oncology indications, and asked panelists to weigh in on why that is the case and how RWD can be used more regularly in trials for common diseases. Julia brought up regulatory and operational challenges in ECA studies in common diseases:
- While regulators now have significant familiarity with ECA studies in rare disease and oncology and they are widely accepted, the acceptability of ECAs for common disease therapeutic areas like cardiovascular and renal has been minimal
- In oncology and rare diseases, clinical trial sizes are typically very small, making data transformation relatively manageable for the ECA, whereas in common diseases there can be thousands of patients, exponentially increasing the complexity of deriving the ECA from RWD
This does not mean common diseases cannot benefit from external controls, however, as Julia pointed back to hybrid-controlled designs, mentioning that regulators are very willing to consider hybrid-controlled designs for common diseases, citing an earlier discussion with FDA leaders.
Science Director
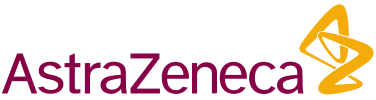
Victoria and Mayur mentioned another approach, RWD-powered covariate adjustment, that has been viewed by regulators as acceptable in common diseases in their discussions. RWD-powered covariate adjustment increases the statistical power to detect a significant treatment effect by using RWD to adjust for the effects of confounding factors on the outcomes. This power gain allows for a reduction in both the treatment and control arm sample sizes required to detect a significant treatment effect without directly replacing patients with external controls. However, Mayur noted that it is still critical to demonstrate the reliability and relevance of the RWD used for covariate adjustment for the trial.
Key takeaways
Processes and infrastructure for RWD submissions
Regulators are demanding relevance and reliability of any RWE used in regulatory submissions, but sponsors’ approaches so far have been mainly ad hoc processes developed to support specific programs and projects, which are often unable to deliver the rigor that FDA is looking for. In this segment, panelists discussed practical approaches and infrastructure to support RWD/RWE submissions.
How does pharma operationalize RWD submissions?
Mayur asked Victoria to describe the practical experience of how Pharma teams organize processes and infrastructure for submissions using RWD for FDA review.
Victoria highlighted the critical role of internal collaboration in pharma RWD/RWE projects. At Amgen’s Center for Observational Research, she described how teams from biostatistics, programming, clinical development, and regulatory affairs work together to ensure alignment within the organization and with regulatory agencies. Mayur added that even convincing regulatory affairs teams internally, before approaching regulators, is a rigorous process in itself. Victoria also mentioned that they request meetings early on in projects with the regulators to talk about the data and how it should be submitted to the agency.
Julia and Hongwei concurred that regulatory submissions with RWD cannot be achieved through an ad hoc process as many studies are performed today. Mayur emphasized that RWD/RWE studies require a highly coordinated, transparent, traceable, and scalable process and infrastructure, just as has been already established for clinical trials for decades, to ensure data reliability and the ultimate validity of the evidence generated using RWD.
What are some practical strategies to solve data relevance issues?
Bringing the discussion back to relevance, Mayur asked the panelists if in addition to using a commercial vendor for the ECA, what if using a pragmatic trial design approach, the ECA patients came from same trial sites as the CT patients - what would that solve regarding relevance and what would it not solve?
Hongwei responded that there would be several benefits from getting the RWD from the same trial sites, including:
- Significant reduction of variability in geography, institution type, and care standards, resulting in a high degree of relevance of the RWD
- Opportunities to continue to collect long term outcomes over the product lifecycle, which is especially important in chronic diseases, providing information on hospitalizations, readmission rates, and other critical metrics after the main trial completes
- Access to RWD at the same trial sites can be very helpful in planning upcoming trials, giving the opportunity to better understand the broader population at the sites to fine tune inclusion/exclusion criteria and estimate enrollment rates
Victoria referenced a previous trial for Amgen’s Blincyto in which the ECA data was collected from many of the same clinical centers in US and Europe to support an indication for relapsed refractory acute lymphoblastic leukemia (ALL). In this case, leveraging trial sites for the ECA led to being able to demonstrate to regulators the comparability of the RWD and CT data in terms of populations, the standard of care, how the data were collected, and how the outcomes were defined.
Victoria noted however that there can be challenges that depend on the indication, giving an example from another project focused on solid tumors in which there was much more variation in care standards across different care settings. To address this issue, Victoria’s team submitted data from both community oncology sites and academic medical centers in order to capture a sufficiently representative and comparable RWD.
for Observational Research
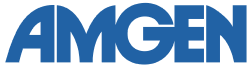
What data standards need to be used for submitting RWD to FDA?
Mayur then turned to data standards, mentioning that the FDA has made it clear that any RWD being submitted to FDA must be in CDISC SDTM. He asked Victoria how Amgen has been implementing SDTM for RWD operationally and how much effort it takes.
Victoria shed light on the operational challenges of converting RWD to CDISC standards, explaining that extensive programming hours are required. It was also noted that converting RWD to SDTM for even just a few hundred patients can take thousands of hours which is nearly tenfold the time typically required for clinical trial data, owing to the scale of variety and dimensionality in RWD that is orders of magnitude higher than clinical trial data. This massive amount of effort stems from the fact that SDTM was designed for RCT data, not RWD. Mayur mentioned CDISC and Droice Labs have partnered to fix this issue through Droice technologies.
for Observational Research
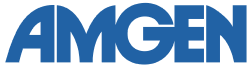
Key takeaways
Summary
Mayur concluded the panel discussion by emphasizing the importance of "fit-for-purpose" data to be able to use RWD for generating primary evidence. Increasingly stringent FDA guidelines require not just justification but quantifiable evidence of relevance, data quality, and traceability. This is evident in the recently released FDA final guidance document on the use of EHR and claims data in regulatory submissions, which raises the bar for scientific rigor in the requirements for demonstrating relevance and reliability of RWD and the validity of the generated evidence.
Mayur further elaborated that the operational difficulties in delivering reliable RWD/RWE stem from the current ad hoc process and emphasized that implementing tooling and infrastructure layers is crucial for ensuring RWD reliability end-to-end, from recruiting CT patients and extracting RWD from multiple study sites though generating the SDTM. Droice Labs is working with multiple Pharma to develop tools and infrastructure for working with RWD that ensures the necessary rigor and scalability of the end-to-end process for generating reliable RWE. The overall panel conversation underscored that achieving fit-for-purpose RWD for regulatory use is a fundamentally different and more complex task than traditional data analysis in RCTs.