FDA final guidance on real-world data (RWD) emphasizes the requirement for data reliability when using RWE in regulatory decisions. Unlike clinical trial data, RWD is never collected in any standard/format. As a result, traditional extract-transform-load (ETL) tools and processes relying entirely on the structure (e.g., schema, data model) of data are inadequate for processing RWD, leading to massive data loss, rendering the RWE unreliable for regulatory use. This inadequacy results in bottlenecks, delays, and rejection by the regulator on data reliability grounds. Droice Labs recently discussed its unique approach for RWD reliability with FDA (see press release) that utilizes both structure and content of RWD for data transformation from various RWD sources—including sites, commercial vendors, registries, and claims—into reliable analysis-ready data as per regulatory requirements. This article outlines Droice’s AI approach and how it is leveraged by multiple major pharma to prepare reliable RWE for regulatory decisions.
The RCT Data Journey
In randomized controlled trials (RCTs), the process of transforming participant data into the study data model or data standard—such as SDTM—is well established and standardized. The journey begins at the point of data capture, where the Case Report Form (CRF) serves as a uniform data capture tool across all study sites. This standardization ensures that data is collected consistently, minimizing variability from the outset.
Following data entry, the CRF data undergoes source data verification, in which study staff rigorously review CRF entries to confirm that the data is accurate and complete. This step is crucial to ensure that the recorded information faithfully represents the participant’s variables like demographics, baseline covariates, exposures, and outcomes.
Once validated, the CRF data is methodically transformed into a predefined standard. Specific transformation rules and mappings convert the raw CRF entries into a structured format that meets regulatory requirements. This set transformation process results in a clean, reliable dataset that facilitates effective analysis and supports regulatory decision-making. The figure below illustrates this RCT data journey.
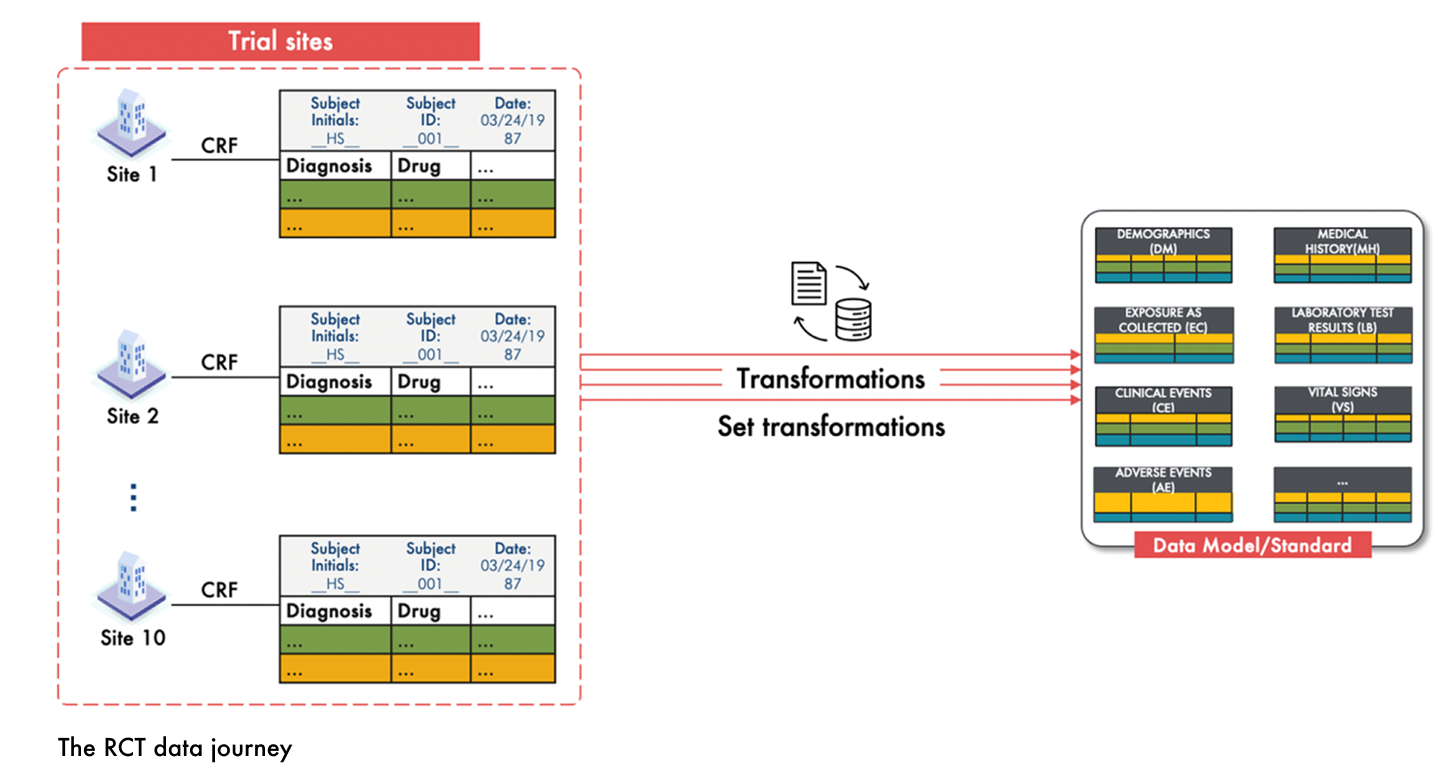
Now, consider a scenario where each site uses its own unique CRF for data capture. Without a uniform CRF across sites, data is collected in different formats, leading to inherent inconsistencies. Instead of following a single, well-defined transformation process, each site's data requires its own unique set of transformation rules and mappings. As illustrated in the figure below, this results in multiple, site-specific workflows that complicate the overall process, increase the potential for error, and challenge the reliability of the final dataset.
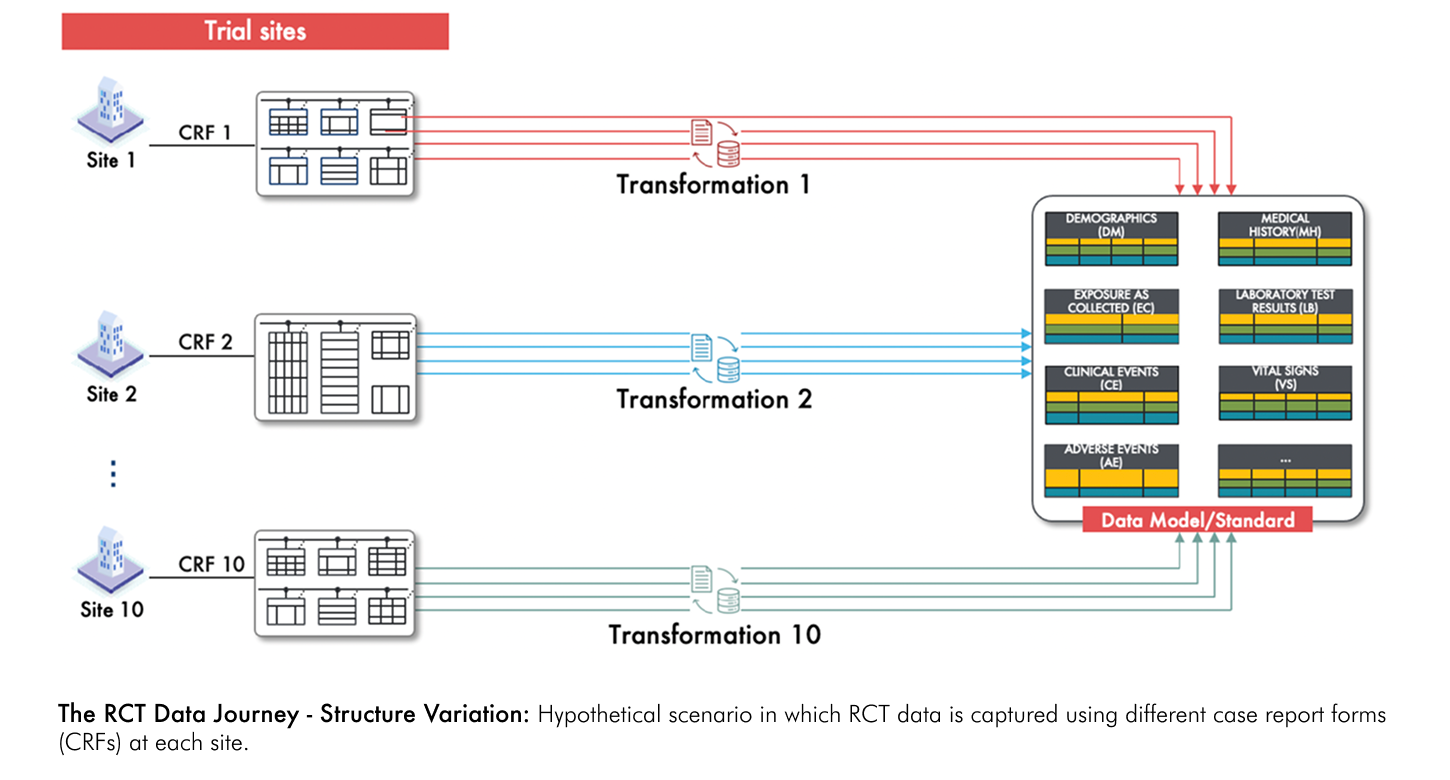
Now, consider a further complication: imagine that outcome data is not recorded consistently in specific dedicated fields of the CRF for each participant but is instead is uniquely dispersed across multiple fields in inconsistent patterns that vary across different participants (see figure below). For example, what if an outcome based on a change from baseline in a particular lab value was found in a field intended to capture a given participant’s medical history? And what if now multiple study variables, not just outcomes, were scattered uniquely in the RCT data for every participant? This would make the transformation process exceedingly complex. Rather than applying a single set of transformations, unique mappings and transformations must be developed for nearly every single participant. This requires a detailed review of every data point for each individual to accurately capture and standardize the variables. Finally, imagine if you had to apply this same intricate, participant-specific transformation process to data from 100 to 10,000-fold more participants than are typically enrolled in RCTs – this would be a nearly impossible task. This complex and labor-intensive process would dramatically increase the risk of error and compromise the reliability of the data.
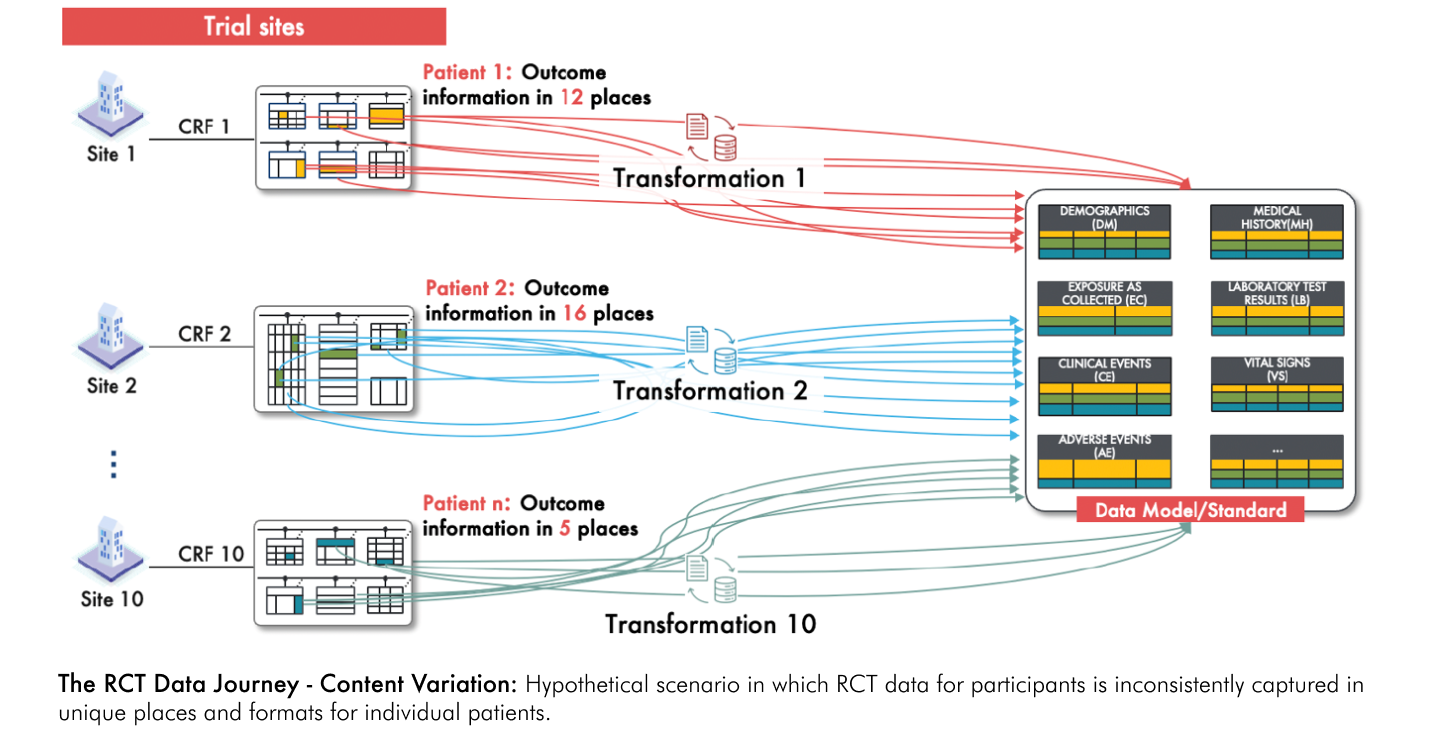
Thankfully handling such messy and inconsistent data patterns on a per-participant basis is not actually the case when conducting RCTs. However this is precisely the challenge when using real-world data (RWD) to generate evidence of treatment efficacy and safety. RWD has massive variability in both structure and content, and is usually orders of magnitude larger than typical RCT datasets (see figure below). This means that the labor-intensive, patient-specific transformation process described above is a reality that must be dealt with in order to use RWD reliably for evidence generation.
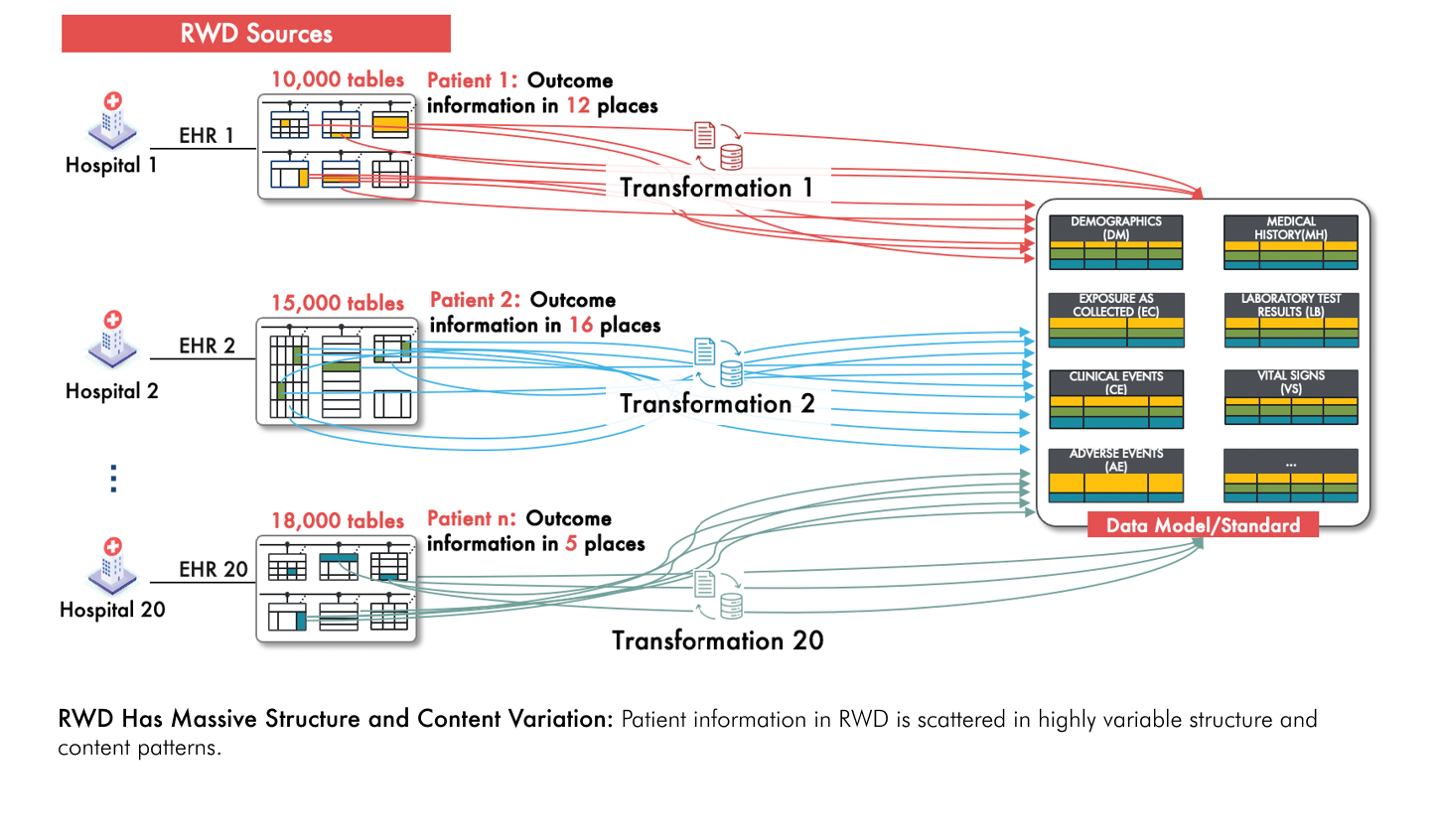
Droice Labs Technologies: Solving RWD Reliability Challenges with AI
To completely and accurately capture patient data from RWD, imagine a process where an expert in the relevant clinical domain—one who also understands the intricacies of complex RWD data structures and the clinical practice nuances of real world settings—meticulously reviews every single data point to identify and extract the study variables. While such a manual process would be prohibitively labor-intensive and error-prone given the volume and diversity of RWD, this is precisely what an AI-driven approach can achieve at scale (see figure below).
Droice Hawk is an AI middleware technology specifically designed for this purpose. Trained on vast amounts of RWD from diverse global sources, it comprehensively scans both the structure and content of the data to accurately identify and extract patient variables from heterogeneous and unstructured RWD sources. Droice SuperLineage provides comprehensive traceability for all source data elements—a critical FDA requirement for data reliability and validation.
In a recent discussion with the FDA, Droice Labs discussed how this AI approach ensures lossless, reliable transformation of RWD into study data. Because Hawk comprehensively processes the data without omitting any patient information, it effectively eliminates information loss from the original source. This lossless approach is critical for ensuring that RWD meets FDA’s reliability standards required for regulatory decision-making.
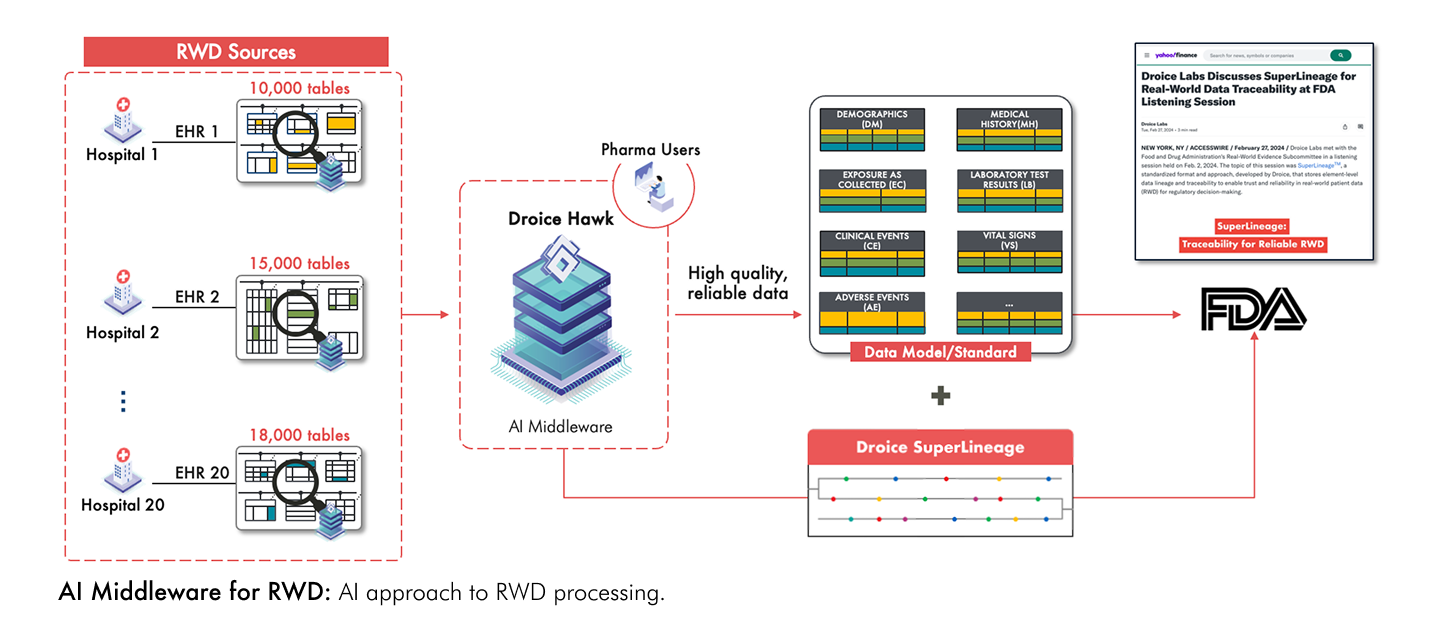
Several big pharma are leveraging Droice’s AI middlewares to transform diverse, global RWD sources into high-reliability, analysis-ready study data for both regulatory and non-regulatory use cases (see figure below). This approach offers several key benefits:
- Reliability: Lossless and comprehensive capture of patient source data with full traceability
- Speed and scalability: Automated identification and transformation of patient data across large volumes of diverse RWD to supporting efficient, timely insights and decision-making
- Fit for regulatory use: Meets rigorous standards required for regulatory submissions to health authorities
- Privacy compliance: Meets stringent data privacy compliance requirements including HIPAA, GDPR, and state/local laws
Using these technologies, pharma companies are fully realizing the potential of RWD to accelerate their research and clinical development timelines.
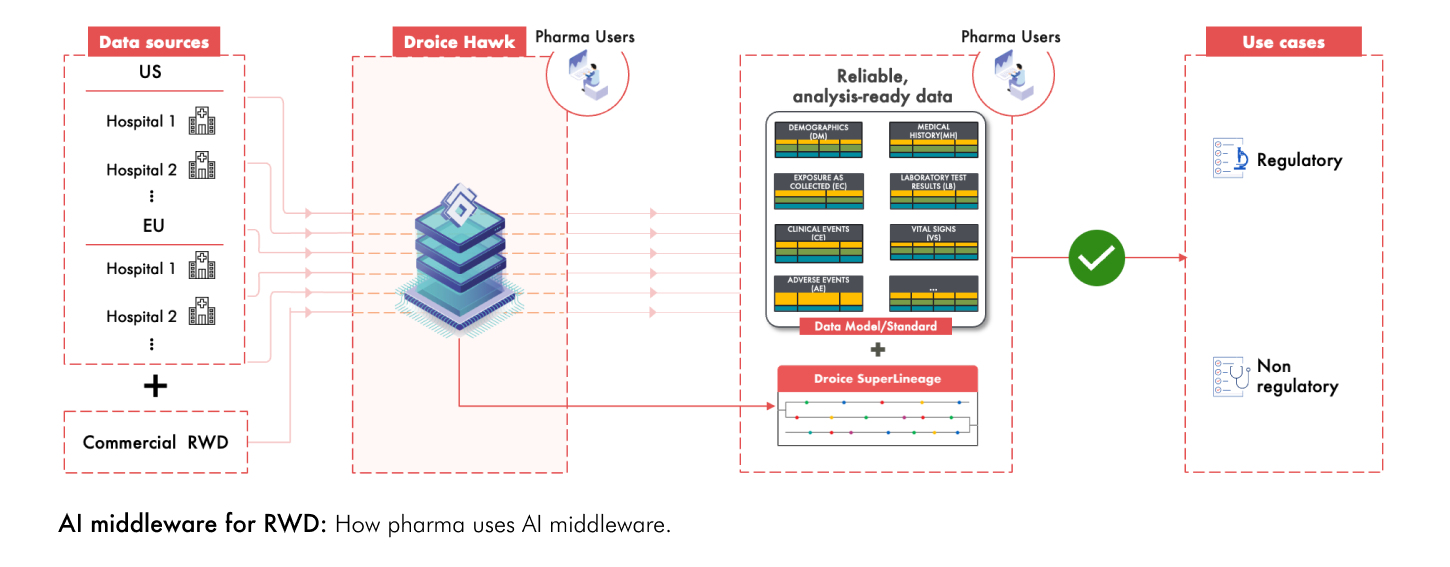
To learn more about how Droice technologies are supplying enhanced reliability, scalability, and robust privacy compliance to enable life sciences companies to accelerate trials for regulatory submissions, please visit www.droicelabs.com.