Healthcare systems continuously generate vast amounts of real-world data (RWD) that contain a wealth of information on patient disease manifestation, progression, and responses to treatment. When appropriately leveraged for research, RWD sources like electronic health records (EHR) and insurance claims offer a gold mine of insights into treatment efficacy and safety in everyday clinical settings. With regulatory bodies like FDA increasingly supporting the use of RWD as primary evidence in submissions, there is a significant opportunity to leverage these data sources to reduce required enrollment in clinical trials and bring life-changing therapies to market faster.
To use RWD in regulatory submissions, FDA currently requires conformance of RWD to Clinical Data Interchange Standards Consortium (CDISC) standards. For sponsors submitting RWD to FDA to support substantial evidence of intervention efficacy and safety, this means transforming RWD into the CDISC Study Data Tabulation Model (SDTM) and the Analysis Data Model (ADaM). However, because RWD is collected for clinical care, there is a complete mismatch between how RWD is organized and represented and how it needs to be structured for a study and represented in SDTM/ADaM for the specific regulatory purpose at hand. As a result, the RWD needs to be extensively transformed and mapped into SDTM/ADaM, which poses major challenges for data reliability. This article examines the reasons why RWD in SDTM/ADaM is by default too unreliable for regulatory decision-making and how it can be made to be reliable.
Why RWD in SDTM is Unreliable
The SDTM/ADaM standards are designed to capture patient-level data for a specific study, encompassing study variables like inclusion/exclusion criteria, arm assignments, exposures, covariates, and outcomes. These variables define the study context and serve as the foundation for all downstream analyses. In clinical trials, these study variables are fully specified by the study protocol, meticulously collected into standardized forms in electronic data capture (EDC) systems by research staff, and harmonized according to the CDISC Clinical Data Acquisition Standards Harmonization (CDASH) guidelines. This process allows for a straightforward transformation of the collected data into SDTM/ADaM following well-established Implementation Guides (IGs), and leads to very high reliability of the SDTM/ADaM data in clinical trials.
In RWD however, there are no explicitly defined study variables like arm assignments, baseline covariates, or follow-up outcomes; these must be derived algorithmically from the original RWD. These derivations involve identifying relevant raw clinical data points (like diagnosis codes, medication records, or lab results) and applying logic to calculate the resulting study variables. The challenge here is that this process is inherently far more complex than the straightforward data collection and mapping processes used in clinical trials. RWD sources like EHR are notoriously noisy, highly diverse, and extremely complex, with no consistent structure across different RWD sources. In fact, each individual patient will have a unique way in which their particular clinical information is represented. This means that transforming and mapping diverse RWD to SDTM/ADaM is an enormous task. The army of well-intentioned data engineers, clinical experts, and epidemiologists that work diligently to transform these data for a single project will still find that this process results in data loss and errors that render the data unreliable.
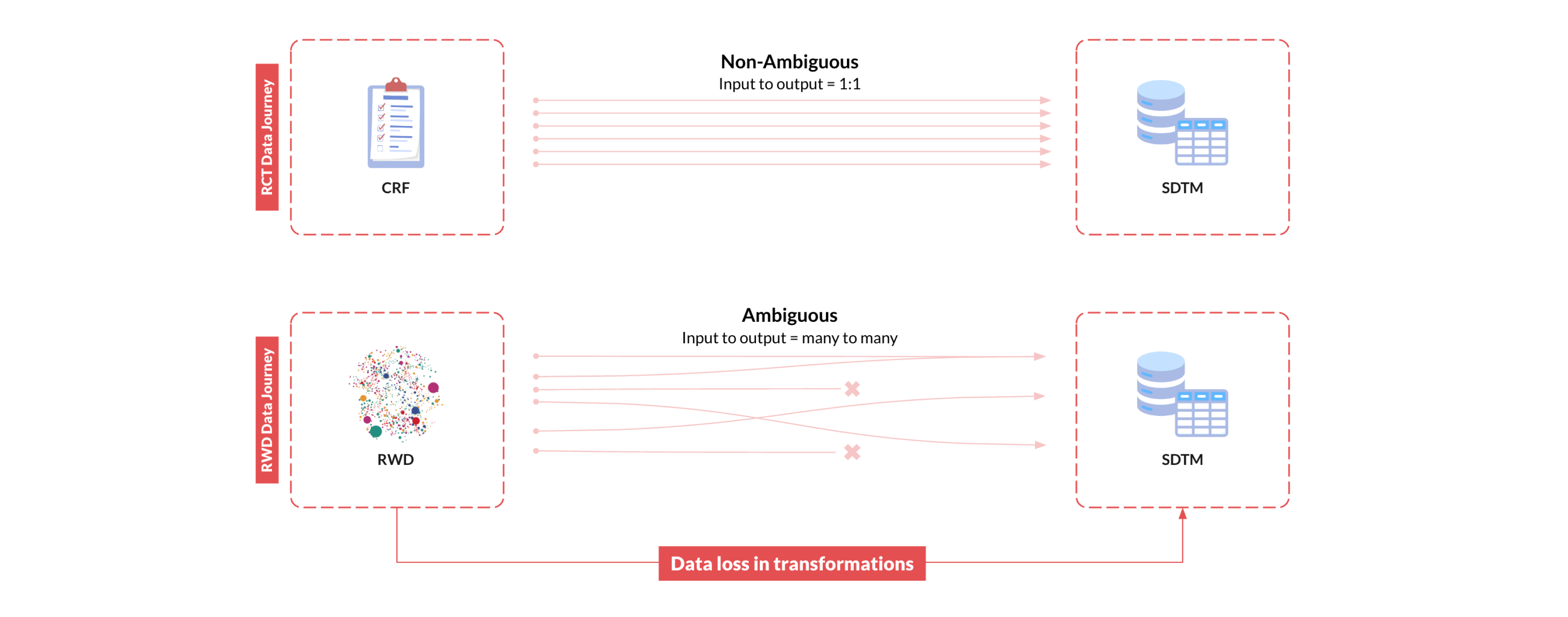
Taking RWD to SDTM/ADaM is not only slow, iterative, and resource intensive, these unknown errors and information loss that occur in the process of generating the RWD-derived study data introduce unquantifiable biases that ultimately undermine the validity of research findings, rendering the RWD unsuitable for regulatory decision-making.
Making RWD in SDTM/ADaM Reliable: Droice Hawk and SuperLineage
To maximize the reliability of RWD from a given source, it is critical to both minimize information loss and error and to have the capacity to measure the accuracy and completeness of the RWD in SDTM/ADaM such that the impacts of error can be taken into account. Droice Labs has developed AI middleware technologies designed specifically to address the data reliability challenges involved in processing and working with RWD scalably for applications demanding the utmost scientific rigor, including RWE generation for regulatory submissions. Droice Hawk is an AI middleware that scalably converts raw, messy RWD into cleaned, harmonized data that massively simplifies the transformation of RWD into high-quality SDTM/ADaM. Droice Hawk’s lossless, comprehensive, and automated processing of source RWD maximizes the accuracy and completeness of patient information extraction and minimizes human error in the process to generate SDTM/ADaM from RWD.
However, even when high-quality RWD in SDTM/ADaM is achieved, it’s not actually reliable unless it is traceable to the source data and its accuracy and completeness can be measured. This is exactly what Droice’s traceability solution, SuperLineage enables. SuperLineage provides various metadata components required by FDA for RWD, including comprehensive data reliability assessments at a data point-level (read about Droice’s discussions on SuperLineage with FDA). SuperLineage achieves this while fully complying with stringent privacy regulations including HIPAA and GDPR — By maintaining comprehensive, data point-level lineage to each source data point for each patient in the study in a standardized format across all sources, SuperLineage enables RWD in SDTM/ADaM to be scalably validated to measure the performance of the algorithmic derivations for each study variable. Quantifying this error bound allows for the potential impact of information loss on efficacy and safety inferences to be quantitatively assessed such that the study inferences can be trusted in the face of these errors.
Droice-CDISC Collaboration
CDISC has been collaborating with Droice Labs since 2022 on projects developing data standards to support the regulatory use of RWD. Through this collaboration, Droice had demonstrated to CDISC its SuperLineage RWD traceability technology and how it supports validation and audit activities for regulatory submissions of RWD. This prompted CDISC to initiate the RWD Lineage project to develop a CDISC standard for RWD lineage metadata for use in submissions, which Tasha Nagamine, CTO of Droice Labs, is spearheading along with Anita Umesh from Roche. SuperLineage is serving as an implementation of this proposed RWD Lineage standard. Solving RWD traceability challenges in a standardized and scalable manner with this approach represents a critically important step in enabling RWD used in submissions to meet FDA’s requirements for RWD reliability for use in regulatory decision-making.
Read about the CDISC RWD Lineage Project.